Inspired by a challenge raised by Prof. Jean-Pierre Hickey in Mechanical Engineering I have worked to investigate novel ways to use Deep Neural Network to revolutionize the way combustion simulation calculations are computed and stored.
This research is relevant for the design of combustion engines and energy production.
Our results are significantly better than existing approaches and could lead to an order of magnitude speed up in some combustion simulation models. This work was presented at the European Conference on Machine Learning conference in September 2019.
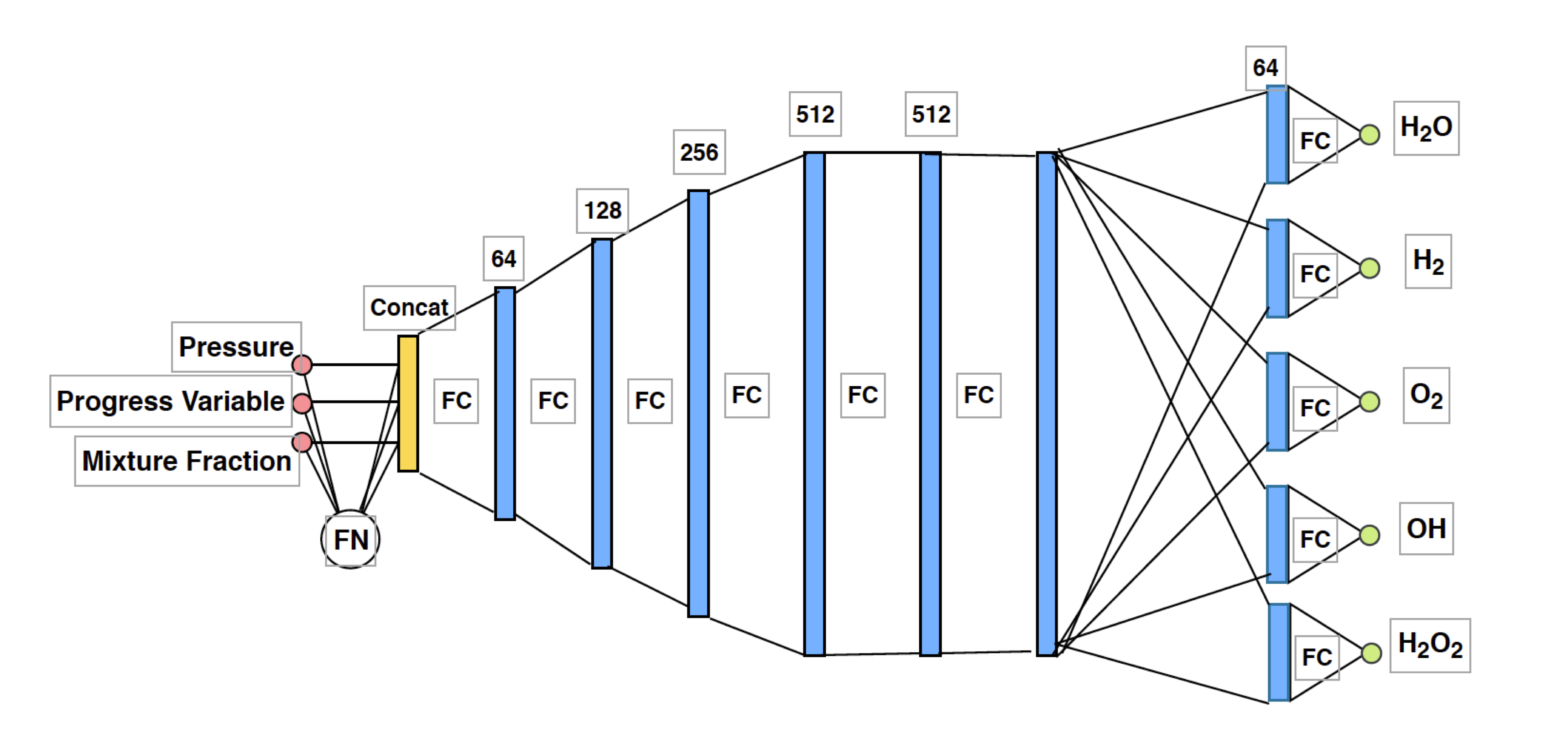
Our Papers on Combustion Modelling
Compact Representation of a Multi-dimensional Combustion Manifold Using Deep Neural Networks
Sushrut Bhalla,
Matthew Yao,
Jean-Pierre Hickey,
and Mark Crowley
In
European Conference on Machine Learning (ECML-19).
Wurzburg, Germany.
2019.
The computational challenges in turbulent combustion simulations stem from the physical complexities and multi-scale nature of the problem which make it intractable to compute scaleesolving simulations. For most engineering applications, the large scale separation between the flame (typically submillimeter scale) and the characteristic turbulent flow (typically centimeter or meter scale) allows us to evoke simplifying assumptions–such as done for the flamelet model to precompute all the chemical reactions and map them to a low order manifold. The resulting manifold is then tabulated and looked up at runtime. As the physical complexity of combustion simulations increases (including radiation, soot formation, pressure variations etc.) the dimensionality of the resulting manifold grows which impedes an efficient tabulation and look up. In this paper we present a novel approach to model the multidimensional combustion manifold. We approximate the combustion manifold using a neural network function approximator and use it to predict the temperature and composition of the reaction. We present a novel training procedure which is developed to generate a smooth output curve for temperature over the course of a reaction. We then evaluate our work against the current approach of tabulation with linear interpolation in combustion simulations. We also provide an ablation study of our training procedure in the context of overfitting in our model. The combustion dataset used for the modeling of combustion of H2 and O2 in this work is released alongside this paper.