Forest Wildfire Management
Wildfire management is the study of managing, predicting, and mitigating risk of forest wildfires.
DOMAINS | forest-management | forest-wildfire | computational-sustainability
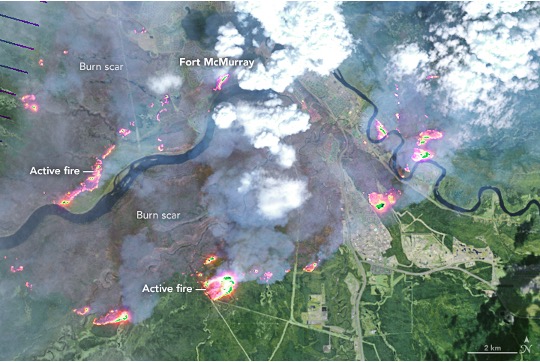
As part of the more general domain of Sustainable Forest Management, we focus on the task of Forest Fire Management which presents a number of unique challenges which push the boundaries of what is possible with existing AI/ML algorithms. These include the importance of considering:
- predicting daily fire spread from historical data automatically by combining information from weather, satellite hotspot detection and other available GIS information
- multiple spatial-temporal scales at all times
- tradeoffs between individual and social good
- paucity of supervised training data
- coordinating decisions amongst a large number of agents (distributed nationwide) without frequent, extensive communication.
Relevant work to this project has been progressing recently on theoretical models of multi-agent decision making. This research explores the intersection of theory in the field of Game Theory and Multi-Agent Reinforcement Learning (MARL), both of which study how multiple agents can adapt and optimize their decision making policies in response to changes in the world around them and actions of other decision makers.
News
- Lab News Dec 12, 2022 : Attended Forest Wildfire Conference on this topic.
- Lab News March, 2022 : Two papers related to this project discussed.
- Lab News Dec 1, 2022 : In Fall 2022, members of the lab went to a Canadian Wildfire conference. Mark also gave a talk on research related to this topic.
- Journal paper in JAIR on a new algorithm using forest widlfire as one of its motivational examples to demonstrate the difficulty of multi-agent planning in real-world domains with many agents acting at once (Ganapathi Subramanian et al., 2022).
- ML for Forest Fire Journal Paper: In 2020, in collaboration with some other investigators in this strategic network, we published a review article in the Environmental Reviews journal (Jain et al., 2020) analysing the relevance of various ML algorithms to the domain and exhaustively surveying and analysing the existing research using ML for forest fire management.
- NSERC/CANADA WILDFIRE STRATEGIC NETWORK: In 2019, an NSERC Strategic Network grant was confirmed in which we are involved to support computational research in to Forest Fire Management practices. This includes the AI/ML/RL focus of our research along with our colleague in computer science Prof. Kate Larson.
- In 2019, we were awarded a computing grant on the topic of Wildfire management: Disaster response, climate change” from Waterloo Artificial Intelligence Institute (WAII) in affiliation with the Microsoft “AI for Social Good” program.
Our Papers on Forest Wildfire Management
- Multi-Advisor-MARLLearning from Multiple Independent Advisors in Multi-agent Reinforcement LearningIn Proceedings of the 22nd International Conference on Autonomous Agents and MultiAgent Systems (AAMAS). International Foundation for Autonomous Agents and Multiagent Systems (IFAAMAS), London, United Kingdom. Sep, 2023.
- Learning Forest Wildfire Dynamics from Satellite Images Using Reinforcement LearningIn Conference on Reinforcement Learning and Decision Making. Ann Arbor, MI, USA.. 2017.